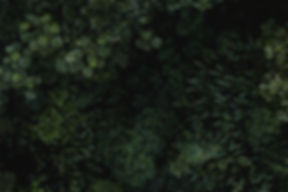

A little bit about me
The tough part about being a mathematical biologist is that the mathematicians think you've gone soft and the biologists think you've gone crazy.
I'm a mathematical biologist. I love developing novel mathematical tools motivated by biological problems, and I love using mathematics to interrogate the logical consequences of biological theories for insightful study design.
To me, the world is a fascinating jungle defined by species (categories of things) interacting, innovating, and evolving. In ecological systems, species compete for resources that arrive at unpredictable times and rates, innovating strategies and the population of newly innovated strategies evolves in a manner determined by competition. In economic systems, companies compete for revenues & investment capital, merge and acquire other companies & assets, innovate new products and business strategies, and expand to new markets. From ecological to economic systems, the living world is a jungle and I try to be its mathematical Tarzan, formally studying those core processes of competition, innovation, and evolution.
I study the jungle by swinging from one vine to the next, studying mathematical principles of species interactions, innovation, and evolution. My work consequently spans many subfields of biology, sociology, economics & mathematics that operate by similar processes. I've published papers on epidemiology, community ecology, community assembly, evolution/co-evolution, stochastic processes, and analyses of financial markets. While I grew up a biologist, I'm growing deeply interested in human systems, such as financial markets and human memetic communities (the ideas floating around in our heads) as innovating and evolving systems similar (but not identical) to biological systems.
I also like to do outdoor sports, philosophize, sip whiskey by the fire, brainstorm cool business ideas (disruptive innovations in this jungle), play with dogs, and read great books.
